The role of data representation for the design, development and evaluation of (medical imaging) AI innovations in healthcare
By Claudia Lindner
Senior Research Fellow, Division of Informatics, Imaging & Data Sciences, University of Manchester
The development and implementation of artificial intelligence (AI) technologies in various fields have seen massive advancements in recent years. In the healthcare sector, the transformative impact of AI innovations is on the horizon – with opportunities to enhance diagnostic accuracy, streamline treatment processes, and improve patient outcomes. With the increasing presence of AI technologies, there is a growing awareness of the importance of data quality for the success and reliability of AI innovations in healthcare.
In the field of computer science, the phrase 'garbage in, garbage out' is commonly used to express that the quality of the output is determined by the quality of the input. For developing AI innovations, this is often linked to the accuracy, consistency and volume of the data. However, even large amounts of accurate data may not be sufficient to develop reliable AI innovations in healthcare if the data are not representative. For example, depending on the overall aim, a technology developed just on data of men may not perform well for women, a technology developed just on data of one ethnicity may not perform well for another ethnicity, and a technology developed on data of adults may not perform well for children (Figure 1). However, data representation does not only refer to the data that are used for training or evaluating the AI technology. The importance of data representation also applies to the broader context in which such data are operating: Where is that data coming from? How has it been generated? How is it being used?
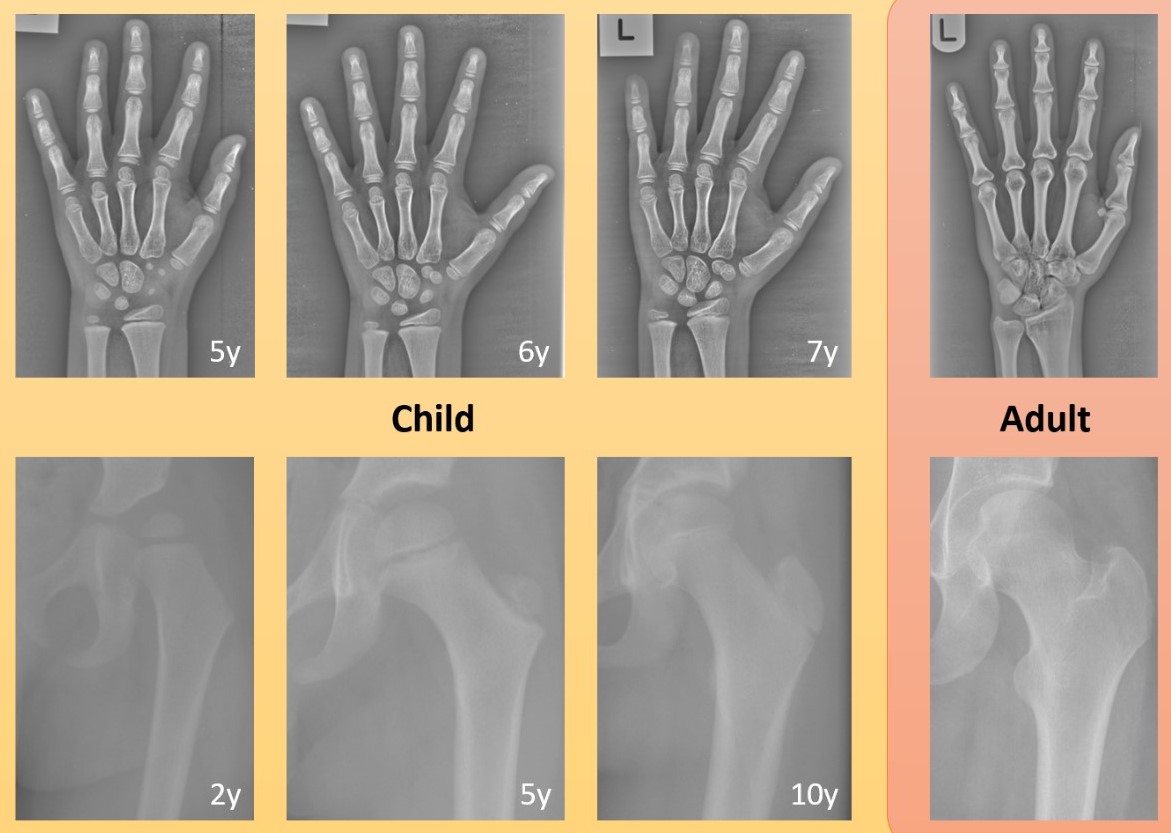
Figure 1. Examples of the developing bones in children over time compared to the same bones in adults: (top) hand x-ray images of a child aged 5, 6, 7 years and an adult; (bottom) hip x-ray images of a child aged 2, 5, 10 years and an adult. Images were cropped and contrast-enhanced to improve visibility. Bones fusing and new bones appearing in the growing child may pose a significant challenge to AI technologies that have been developed using adult data only.
The quality of the data representation directly impacts the effectiveness and safety of AI technologies in healthcare. Understanding the data landscape from the outset is a key requirement for the design, development and evaluation of AI innovations in healthcare. This requires the following considerations:
- Clinical context: Data representation here refers to the understanding of the clinical need as well as of current clinical procedures and workflows to inform the design of the technology to be developed. This does not only apply to the healthcare professional perspective but also to the public and patient view on what may be needed and would be accepted. Clinical context is also relevant for evaluating the AI technology so as to ensure that metrics of clinical relevance are applied when assessing performance. In addition to leading to a technology that is needed and performs well, understanding the clinical context will also facilitate the implementation and adoption of the innovation.
- Fairness and bias mitigation: When developing an AI innovation in healthcare there is the potential for harm by introducing bias into the innovation. If the data used to develop the AI innovation does not accurately reflect the diversity of the patient group it is supposed to help, then the AI innovation might end up being unfair or biased. Over the years, there have been a number of examples in the news where AI-based face detectors were affected by bias, for example, with regards to skin colour. In healthcare, a biased AI innovation could have profound consequences, leading to misdiagnoses and compromised patient care. Biases can be mitigated by ensuring that the training data are diverse and inclusive with regards to age, gender, ethnicity, and other factors.
- Generalisability: The training data used for developing the AI innovation will need to be representative, covering a wide range of scenarios. This does not only apply to the characteristics of the population but also to other real-world factors. For example, in the context of medical imaging, this also applies to the scanner, which may influence image quality, as well as the image acquisition protocol, which may influence patient positioning during image acquisition. For electronic health records, this may relate to differences in coding systems, data formats, or documentation styles. The more representative the training data, the more likely the AI innovation will work in multiple scenarios. Similar considerations apply to the testing data used for evaluation as the AI innovation will need to be validated on independent, representative data. This will provide evidence of reliable performance in real-world applications, and availability of such evaluation results will contribute to building trust in the AI innovation, a prerequisite for clinical acceptance and adoption.
When entering new collaborations for developing novel systems to outline structures in medical images, we are often asked “How much training data are needed to generate a well-working system?” and the answer tends to be “Well, it depends”. It depends on how representative the data are. A big dataset of largely the same data (e.g. only including images/data of a single scanner, gender, ethnicity) may not be as useful as a smaller dataset that better reflects the real-world variation. The question should not be “How much data?” but rather “What data?”, the answer to which will require a thorough understanding of the clinical application at hand. For example, for a medical imaging system aimed at a particular body part and medical condition, this requires an understanding of the anatomical variation of that body part across the target population as well as any variation caused by the medical condition – all of which should be represented in both the training and validation data.
With regards to AI solutions in healthcare that are already available, there is evidence that the above is not always adhered to. For example, it has been shown that even among regulatory approved AI innovations only a minority have peer-reviewed evidence of performance, in particular external validation studies are lacking1,2. The Health AI Register is a very useful resource to find out more about the specifications and evidence of performance for AI-based radiology solutions, including for a range of musculoskeletal applications.
In conclusion, the advancements in AI technologies within healthcare offer promising transformations, but their success depends on the quality and representativeness of the utilised data. A thorough approach to data representation is essential for creating ethically sound, clinically relevant, and widely accepted AI solutions in healthcare.
References
- van Leeuwen KG, Schalekamp S, Rutten MJCM, van Ginneken B, de Rooij M. Artificial intelligence in radiology: 100 commercially available products and their scientific evidence. Eur Radiol. 2021;31(6):3797-804.
- Kelly BS, Judge C, Bollard SM, Clifford SM, Healy GM, Yeom KW, Lawlor A, Killeen RP. Radiology artificial intelligence: a systematic review and evaluation of methods (RAISE). Eur Radiol. 2022;32(11):7998-8007.