Developing AI for healthcare: where, why and how to do it in a responsible way
Author: Justin Green
Developing AI for healthcare: where, why and how to do it in a responsible way
Justin Green is an early year’s orthopaedic registrar who has been involved in clinical informatics and AI innovation within healthcare. He is involved as the Clinical Data Science and Technology Lead on OpenPredictor Project where Justin focuses on developing robust and responsible innovation of clinical decision systems using AI and machine learning.
What is machine learning (ML)
Technology in healthcare seems to be evolving at an ever-increasing rate and two terms, artificial intelligence (AI) and machine learning (ML), are becoming far more common phrases in discussion of medicine than ever before. These two terms are often used interchangeably and frequently find their way into the discussion on digital transformation and the future of global healthcare. There is undoubtedly potential in these novel approaches and the pace of technological change within healthcare appears to be going in only one direction at speed with little sign of slowing down. But are we taking the right approach to utilising AI in healthcare and are we doing it in a responsible manner? Ensuring we are applying the right technology for what we need, in the right way to give us meaningful outcomes?
AI and ML are subtly different approaches to solving complex problems. AI is the science of generating human-like intelligence to make sense of a situation, while AI makes use of patterns to appear smart; inferring meaning from a passage of text or distinguishing a picture of a person from an animal. ML on the other hand is a far more refined approach to solving complex problems. ML is a subset of AI and a component of the bigger AI system. ML is a powerful technology that enables the ‘machine’ to independently find connections in large datasets by building algorithmic connections to create solutions for predetermined problems. It has evolved from basic pattern recognition and learning without programming to the sophisticated AI-enabled learning we see today. ML enables the computer to generate the decisions that provide results and, with little or no human interaction ML can process massive sets of data and independently find recurring patterns to present solutions to complex problems. The computational power of ML is far in excess of human capabilities, but this capacity to compute is strictly limited to the domain in which it is applied. That is to say, ML may produce astonishing results in identifying bone lesions when shown an x-ray, but present ML with cross sectional imaging and it will become unresponsive.
ML is not a new technology, but it is one that has seen substantial improvements in its capabilities over recent times and is now at the point where it can be used to solve vastly complex calculations in big health data. Through a continuous cycle of learning and technological innovation that strengthens the computations and improves accuracy, results can be obtained far faster and with greater reliability than ever before.
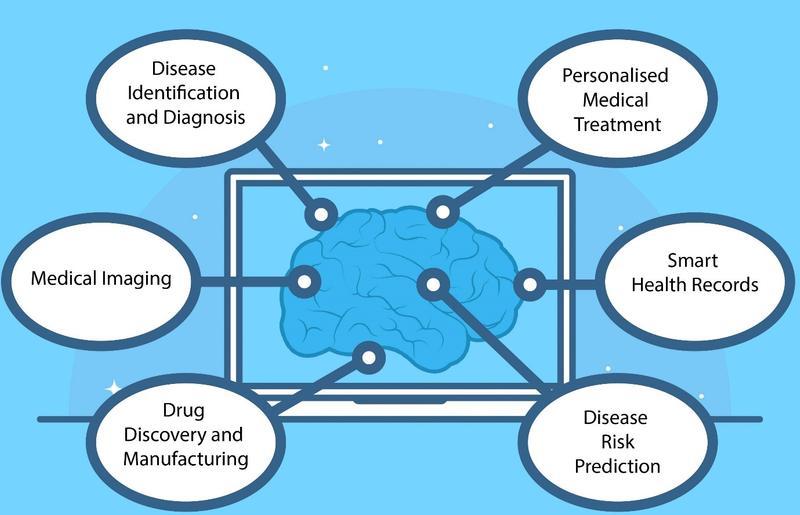
How it can be used
The healthcare sector is leading the way in the adoption of AI technologies and ML is at the forefront of areas such as personalised medicine, prediction into chronic disease progression, developing advanced medical procedures and operational applications.
ML offers a great opportunity to improve health outcomes, access to treatment and operational efficiencies. Take the elective recovery programme for example, as a result of the national measures to cease elective surgery during the 2020 global pandemic, the NHS is facing a crisis in recovering elective procedures as waiting lists continue to increase. At this time, 6.6 million people currently occupy waiting list pathways with a median wait time of 12.7 weeks. Within orthopaedics, 750,334 people are waiting on average over 14 weeks to be seen, of these 55,418 will be waiting over a year1. The OpenPredictor project uses ML to identify individuals suitable for high volume low complexity (HVLC) recovery programmes. Using over 200 patient specific parameters from over 25,000 admission records, OpenPredictor determines the probable outcome from surgery for patients awaiting primary arthroplasty. The risk stratification model is being piloted in the classification of large volumes of waiting list patients into high, normal and low risk. This open source code will support the North East North Cumbria recovery programme and utilise the full potential of HVLC pathways.
Other examples where specialists across healthcare are continuously looking for innovative ways to leverage the benefits that ML can bring to our patients. ML lends itself well to areas such as image analysis. Many will have heard of the InnerEye project, from Microsoft Research Cambridge and Cambridge University Hospitals NHS Foundation Trust. InnerEye, an open-source module which has provided the foundation for computer vision, a tool used in the processing, automated segmentation and assisted diagnosis of medical imaging. The potential uses in trauma and orthopaedic diagnosis and preoperative planning are obvious2.
Taking a responsible approach
As the adoption of AI/ML continues, there is an unquestionable need to ensure that not only the development proceeds in a manner that supports the patient, but also incorporates a responsible and fair approach to using ML. Responsibility in terms of AI/ML is the evaluation and explanation of the whole modelling life cycle. AI has the potential for significant good, but also the real possibility of causing harm, either as an unintended consequence or through design. As we feed in information from electronic health records, ML will identify characteristics in the data that may not be overtly apparent. Although such characteristics provide valuable insights into areas of further research. It is important to recognise the potential inherent disparities that reside in the same data may also become amplified. A recent review demonstrated lower socioeconomic status combined with ethnicity demonstrating increase surgical needs3. Should the data we use not accurately represent ethnicity, the models we produce will result in patterns that over or under estimate the importance of the feature as opposed to utilising the full breadth of information provided, thus potentiating unfair and/or biased results. An approach to mitigating the potential for harm is to utilise tools which incorporate the functional explainability into any modelling approach.
Explainability is process in which transparency is applied to ML modelling. The complexity of algorithms can be generated through ML leaves it impossible to understand how a prediction is determined using the output alone. Using tools that explain the steps taken in identifying the correlated features that lead to the prediction outcomes is the process of applying explainability.
The OpenPredictor project has demonstrated explainability is a fundamental component in responsible AI. Explainability also opens the door to other potential areas of further research. The OpenPredictor modelling indicated blood test values are far superior in determining outcomes than comorbidities alone (Figure 1).
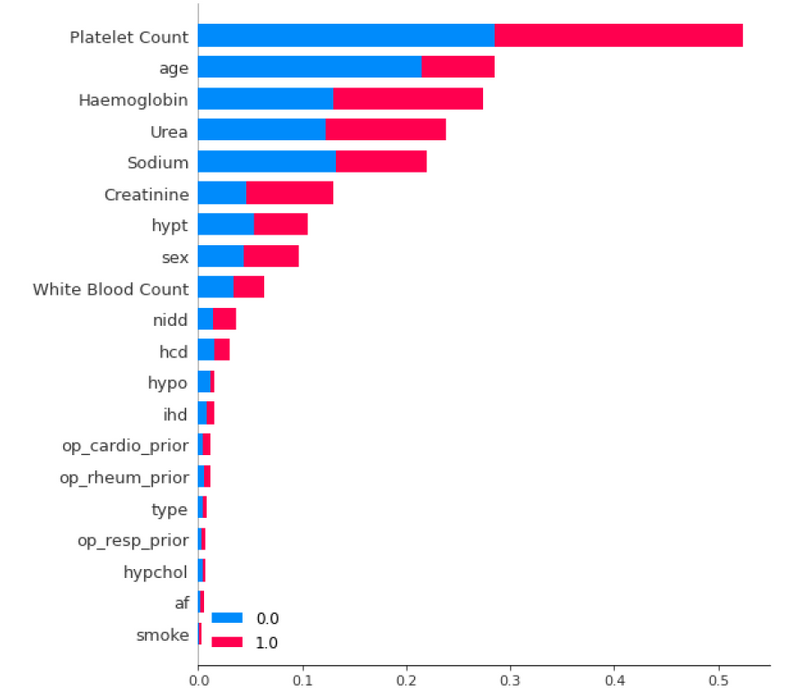
Figure 1: Average impact of input variable on model predictions of successful surgery.
Using explainable ML will indicate correlations in the data however these are not necessarily the causation, rather the features that are most important to the model. Explainability provides the overview, but it must be acknowledged that in the absence of causality or omitted information, ML will seek alternative correlations that may be spurious or indeed, wholly incorrect.
Providing results without transparency compounds concerns in closed and obscure AI systems, the ‘black box’ phenomenon which persist as an understandable barrier to clinical adoption. Simply put, healthcare ML must be handled in an ethical manner.
Required changes
ML should be considered as a powerful tool, but a tool nonetheless. New systems that facilitate low-code programming are making ML more accessible and opening the doors to explore a huge number of possibilities for those who have an interest but not necessarily the technical background. This is surely a win for innovation and will strengthen appreciation and acceptability of all AI technology. As the technology has become more accessible, the data often remains entrenched in antiquated and siloed systems. If we are going to capitalise on ML, there are areas that need to change. As discussed, ML has the capacity to ingest very large datasets, however volume of data alone is not the only answer, the data needs to be pertinent to use case. As we have found in the OpenPredictor project, a large dataset that contains inconsistent or irrelevant information is at risk of generating erroneous results whereas, a smaller dataset that is richer and more balanced in representation will realise far greater accuracies than one that contains massive amounts of vague information. This is something to bear in mind when using electronic health records, the NHS has collected decades of clinically coded information that, for the most part, does not afford the granularity to represent the clinical complexity of a person’s health alone.
Conclusion
AI and ML are a vital evolution in the provision of medicine and healthcare and will become integral tools in our routine practice. Where we will see the most benefit is in the automation of routine and augmentation of care; creating capacity to treat an ever aging population or as we see today, recovering from an excessive backlog in surgery. As the healthcare industry shifts towards this new era of digital transformation, the responsibility will reside with us, as doctors, to provide human agency and to acknowledge the foundation on which it is built. ML alone will not provide all the answers, without topical and rich data it is unlikely that we will derive substantial benefits in addressing the problems to which it is applied. The original question “Are we taking the right approach to utilising AI in healthcare and are we doing it in a responsible manner? Ensuring we are applying the right technology for what we need, in the right way to give us meaningful outcomes?” The answer is ‘we can’.
References
- NHS England. Consultant-led Referral to Treatment Waiting Times Data 2022- 23. Available from: www.england.nhs. uk/statistics/statistical-work-areas/rttwaiting-times/rtt-data-2022-23. [Last accessed 15 July 2022).
- Kurmis AP, Ianunzio JR. Artificial intelligence in orthopedic surgery: evolution, current state and future directions. Arthroplasty 2022;4(1):9.
- Ryan-Ndegwa S, Zamani R, Akrami M. Assessing demographic access to hip replacement surgery in the United Kingdom: a systematic review. Int J Equity Health 2021;20(1):224.