HACKATHON! at the AI in Orthopaedics Conference 2024
Calling all Orthopods!! We are excited to invite you to our ground-breaking upcoming offline Hackathon! This event is a fantastic opportunity for members of the orthopaedic, musculoskeletal and data-science / wider community to collaborate and demonstrate how AI can enhance decision-making in real-life clinical scenarios.
Here’s how it will work
- By Thursday, Novemeber 21, 2024 (6 pm UK time): Interested participants must email their participation details (name, job title, affiliation, and team leader) to [email protected]. No maximum size and one-member teams are welcome, however we would expect a mix of team member skills, with between 2-8 people. At least one team member must register for the conference via the button below. However, we hope that most members of the team will register and definitely benefit by attending the conference.
2. On Friday, November 22, 2024: The Stryker team will share the problem statement, dataset, and its description with the registered participants.
3. By Monday, December 09, 2024 (6 pm UK time): Each team must submit their work via email to [email protected] in the form of a Python script (e.g. Jupyter notebook) and a slide deck summarising their approach and key findings.
4. By Monday, December 16, 2024 (6pm UK time): The Stryker team will select the best solution from all submissions.
5. On Thursday, December 19, 2024: Two teams with the best solutions will present their work during the Stryker hackathon slot at the 'AI in Orthopaedics and MSK' conference.
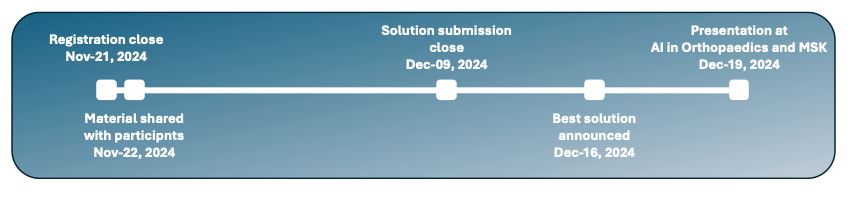
Selection and Judging
Team selections are subject to approval by the BOA and Stryker following your application. On selection, further instructions will be sent by the team.
Submissions will be judged on the following criteria:
- Understanding of the problem statement (1-10)
- Quality of the proposed solution and results (1-10)
- Capability to implement the solution (1-10)
- Bonus challenge (differentiator) (1-3)
The problem statement will involve creating a predictive model based on tabulated data, similar to the approach by Motesharei et al. Predicting robotic-assisted total knee arthroplasty operating time : benefits of machine-learning and 3D patient-specific data.
SUBMIT YOUR TEAM HERE
We look forward to your participation!
Please contact the BOA Events Team if you have any queries via [email protected]